Artificial intelligence (AI) is the use of machines to do tasks that traditionally mandate human expertise and intelligence. Autonomous vehicles are one example, maneuvering the roads without the need of a human driver. The term “artificial intelligence” is often used in tandem with “machine learning,” which is teaching a computer to make a decision based on the troves of data. For example, you might have a computer learn to recognize snow leopards by feeding it a large data set of snow leopard pictures. Every snow leopard the computer categorizes helps make the AI stronger. While still in its infancy, AI is being used in virtual assistants such as Siri and Alexa as well as in the Netflix algorithm, which recommends programming based on user activity. In medicine, AI holds great promise, helping to more reliably develop new drugs, utilize the abundance of medical data, and efficiently assist with diagnostics.
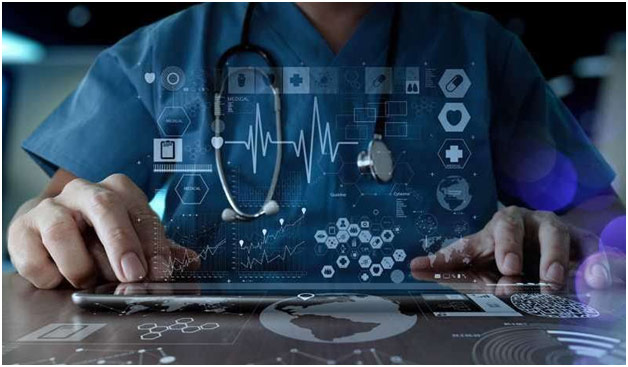
medicine ready artificial intelligence
AI can help revolutionize drug development, a particularly tedious and difficult process where only one out of every 40,000 compounds makes it to market, and getting one drug to market costs $2.6 billion. Atomwise is one company making waves in this space, using AI to predict how a drug candidate will bind to a target protein, being able to scan through billions of candidates before choosing the best one to bring to market. Similarly, biotechnology company Berg is using AI to discover novel ways that cancer cells differ from normal cells. Data is obtained at both a biological (proteins, lipids, etc.) and clinical level before being combined and fed into their basic algorithms to discover previously unknown targets. They can then use basic to find drugs that would help return the diseased cells to the biological levels of the normal cells. Another company, BioXcel Therapeutics, looks to using existing drugs to treat new diseases. Such precedent has already been established with the anesthetic ketamine being now used to treat tinnitus, a hearing disorder, and the infamous drug thalidomide, which was used to treat morning sickness, is now used to treat multiple myeloma. BioXcel Therapeutics leverages AI to screen already approved drugs for new therapeutic targets. For example, they reformulated the IV anesthetic dexmedetomidine for calming patients with bipolar disorder and schizophrenia, for example. Since old drugs have already been FDA-approved, companies can speed up the timetable for bringing the repurposed drug to market. The implications of these companies’ applications of AI are colossal as we can tackle disease more effectively while also decreasing drug costs as less money will be needed to develop pharmaceuticals if drug development is more targeted in nature.
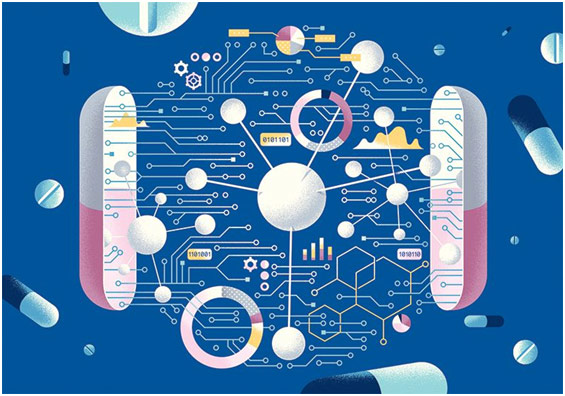
AI in Drug Development
With the troves of medical data in the age of electronic medical records (EMRs), AI seems poised to take that data and help doctors with their decision-making. Beth Israel worked on an AI that could predict which patients were going to miss their doctor’s appointments, and AiCure created an app that captured data from patients to determine if they were adhering to their medication schedules. Considering that missed appointments drain a hospital’s resources and nonadherence costs the nation $300 billion a year, there is an enormous financial benefit to these applications of AI. More importantly though, in both cases, healthcare personnel can intervene, if needed, to counsel the patient, helping to decrease mortality and morbidity. Another company, KenSci, takes in data from various sources, such as EMRs, public records, wearable devices, etc., to predict a patient’s risk for various medical conditions from sepsis to heart attack. Based on what actually happens to the patients, the algorithm is refined so that it becomes increasingly powerful. Considering that chronic conditions burden our healthcare system with the enormous cost of care and repeated hospital readmissions, being able to predict whether a patient is going to have a disease can allow doctors to take important preventive steps in the early stages of the chronic disease.
With AI affording greater insights about each unique person, therapies can also be increasingly personalized to the patient in order to improve treatment efficacy and decrease side effects. Precision medicine is paramount because, for one, most drugs do not work as well on people of color. This travesty can be attributed to the fact that only 5% of medical research participants are people of color while 40% of the US is made up of people of color. With AI, certain drugs can be given to certain people based on their unique genetic and environmental profile. By leveraging patient medical data, we can improve outcomes for patients.
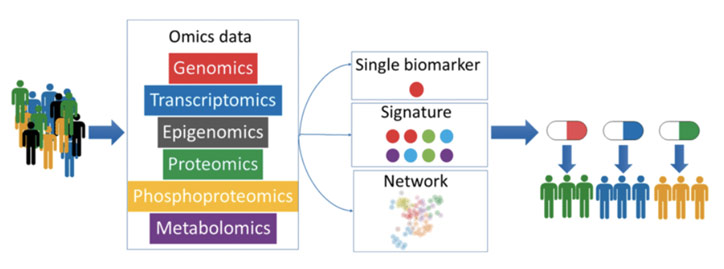
Using AI for Precision Medicine
AI can also be used for interpreting and analyzing imaging data to be able to make a diagnosis with high specificity and sensitivity. For instance, in a match between an AI system and 101 radiologists, the AI outperformed a majority of radiologists when it came to diagnosing breast cancer mammograms. In another study, the ChXNeXt AI algorithm performed as well as radiologists in diagnosing ten different pathologies from chest x-ray scans, even outperforming radiologists for one pathology. That being said, it took over 100,000 x-rays to train the ChXNeXt AI algorithm. For all other types of imaging, the tale is very much similar: machines can read the scans and autonomously make good decisions. Indeed, AI could not only reduce medical errors, which 250,000 people die from each year but also bring greater access to care as any hospital could have access to quality imaging interpretation. The concern here, however, is that AI may devastate the radiology specialty. After all, radiology already has a digital imaging dataset that can easily be fed into an algorithm to train it. The fact that AI has a photogenic memory and never grows tired only adds to this concern.
Nonetheless, there are reasons why that concern may be overblown. There is the issue of who is to blame if an algorithm misdiagnoses a patient, leading to delayed treatment for example. Because of that concern as well as some patient unease with being treated by a computer, a radiologist may always have to independently check the scan as well. Furthermore, if the algorithm makes a mistake, there is no way to determine why it did that because of the black box problem: by design, we cannot discover how the algorithm makes its decision. With AI, we lose the ability to understand why. On top of that, current AI algorithms are quite narrow in what each can identify, and getting the hundreds of thousands of images needed to train them can be difficult given no central repository. In short, there are numerous complications to having AI entirely replace doctors.
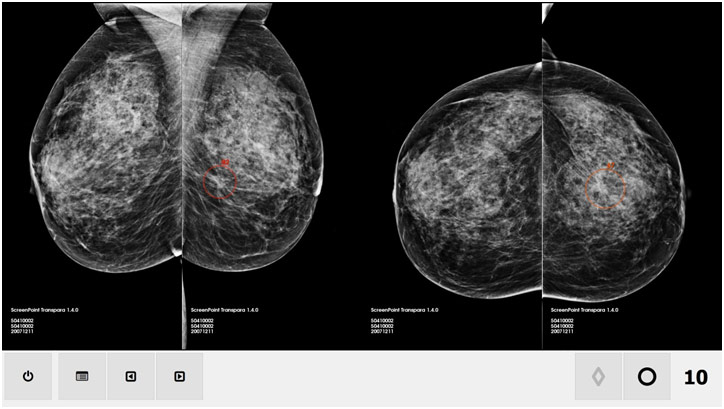
Artificial Intelligence System Scanning Mammograms
Artificial intelligence will dramatically shift medical delivery in the US by freeing doctors and administrators from expensive, burdensome chores. One day, patients might see a kiosk and, based on their reported symptoms and a quick nurse exam, be told by the AI what test (labs or imaging) to get. The AI will then analyze the data and dispense the appropriate medicines or schedule a meeting with a primary care physician or specialist. This AI would be able to free primary care physicians from being inundated with coughs and colds so that they would be better able to treat more serious pathologies. This is only one example of how AI could revolutionize medicine for the better. Indeed, AI will certainly contribute to the ever brightening horizon of medicine’s future.
REFERENCES
Armitage, Hanae. “Artificial Intelligence Rivals Radiologists in Screening X-Rays for Certain Diseases.” Stanford Medicine, 20 Nov. 2018, med.stanford.edu/news/all-news/2018/11/ai-outperformed-radiologists-in-screening-x-rays-for-certain-diseases.html. Accessed 21 Dec. 2019.
“Atomwise, Hanson Pharma Enter into Up-to-$1.5B AI-Based Drug Discovery Partnership.” Genetic Engineering & Biotechnology News, 12 Sept. 2019, www.genengnews.com/news/atomwise-hansoh-pharma-enter-into-up-to-1-5b-ai-based-drug-discovery-partnership/. Accessed 21 Dec. 2019.
Botha, Marc. Artificial Intelligence. Towards Data Science, towardsdatascience.com/artificial-intelligence-is-just-a-tool-aab880f1bbdd. Accessed 21 Dec. 2019.
Davenport, Thomas, and Keith Dreyer. “AI Will Change Radiology, but It Won’t Replace Radiologists.” Harvard Business Review, 27 Mar. 2018, hbr.org/2018/03/ai-will-change-radiology-but-it-wont-replace-radiologists. Accessed 21 Dec. 2019.
Keen, Cynthia. “Artificial Intelligence versus 101 Radiologists.” Physics World, 22 Apr. 2019, physicsworld.com/a/artificial-intelligence-versus-101-radiologists/. Accessed 21 Dec. 2019.
Marconi, Michele. How Artificial Intelligence Is Changing Drug Discovery. 30 May 2018. Nature, www.nature.com/articles/d41586-018-05267-x. Accessed 22 Dec. 2019.
Missakian, Natalie. “BioXcel Therapeutics Unveils New AI Lab.” New Haven Biz, 13 Sept. 2019, www.newhavenbiz.com/article/bioxcel-therapeutics-unveils-new-ai-lab. Accessed 21 Dec. 2019.
Ray, Amit. Precision Medicine. Amit Ray, amitray.com/artificial-intelligence-precision-medicine/. Accessed 22 Dec. 2019.
Rebhan, Andrew. “Medication Nonadherence Is a $300 Billion Problem – Here’s How 3 IT Vendors Want to Solve It.” Advisory Board, 15 Feb. 2018, www.advisory.com/research/health-care-it-advisor/it-forefront/2018/02/medication-nonadherence. Accessed 21 Dec. 2019.
screenprint Medical. The Artificial Intelligence System at Work. Physics World, physicsworld.com/a/artificial-intelligence-versus-101-radiologists/. Accessed 22 Dec. 2019.
Shutterstock. Improving the Quality and Safety of Services Is Inherent Goal of Automation in Health Care. Medical Xpress, medicalxpress.com/news/2018-09-medicine-ready-artificial-intelligence.html. Accessed 22 Dec. 2019.
Shepherd, Ray. “The Third-leading Cause of Death in US Most Doctors Don’t Want You to Know about.” CNBC, 22 Feb. 2018, www.cnbc.com/2018/02/22/medical-errors-third-leading-cause-of-death-in-america.html. Accessed 21 Dec. 2019.
Sullivan, Thomas. “A Tough Road: Cost to Develop One New Drug Is $2.6 Billion; Approval Rate for Drugs Entering Clinical Development Is Less than 12%.” Policy & Medicine, 21 Mar. 2019, www.policymed.com/2014/12/a-tough-road-cost-to-develop-one-new-drug-is-26-billion-approval-rate-for-drugs-entering-clinical-de.html. Accessed 21 Dec. 2019.
Wiggers, Kyle. “KenSci Raises $22 Million for AI that Cuts Health Care Costs.” Venture Beat, 7 Feb. 2019, venturebeat.com/2019/02/07/kensci-raises-22-million-for-ai-that-cuts-health-care-costs/. Accessed 21 Dec. 2019.
“Your Drugs Probably Weren’t Tested on People of Color.” Bright Magazine, 7 Feb. 2018, brightthemag.com/health-medical-research-pharmaceutical-minorities-eugenics-5c5284a8cd7f. Accessed 21 Dec. 2019.
This is good insight for my friends, so I’ll link back to this post and you should likely get some new readers. It’s better than anything else out there when it comes to this topic. Thanks for the fresh viewpoint!